VariationalApplyMPO¶
full name: tenpy.algorithms.mps_common.VariationalApplyMPO
parent module:
tenpy.algorithms.mps_common
type: class
Inheritance Diagram
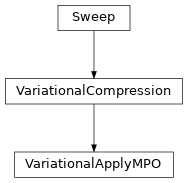
Methods
|
Initialize self. |
|
Perform N_sweeps sweeps without optimization to update the environment. |
Define the schedule of the sweep. |
|
|
Initialize the environment. |
Create new instance of self.EffectiveH at self.i0 and set it to self.eff_H. |
|
Algorithm-specific actions to be taken after local update. |
|
Prepare everything algorithm-specific to perform a local update. |
|
Reset the statistics. |
|
Run the compression. |
|
|
One ‘sweep’ of a sweeper algorithm. |
|
|
|
|
|
Perform local update. |
|
Class Attributes and Properties
|
-
class
tenpy.algorithms.mps_common.
VariationalApplyMPO
(psi, U_MPO, options)[source]¶ Bases:
tenpy.algorithms.mps_common.VariationalCompression
Variational compression for applying an MPO to an MPS (in place).
To apply an MPO U_MPO to an MPS psi, use
VariationalApplyMPO(psi, U_MPO, options).run()
.The goal is to find a new MPS phi (with N tensors) which is optimally close to
U_MPO|psi>
, i.e. it is normalized and maximizes| <phi|U_MPO|psi> |^2
. The network for this (with M tensors for psi) is given by.——-M[0]—-M[1]—-M[2]—- … —-.| | | | |LP[0]—W[0]—-W[1]—-W[2]—- … — RP[-1]| | | | |.——-N[0]*—N[1]*—N[2]*— … —-.Here LP and RP are the environments with partial contractions, see also
MPOEnvironment
. This algorithms sweeps through the sites, updating 2 N tensors in eachupdate_local()
, say on sites i0 and `i1`=`i0`+1. We need to maximize:.——-M[i0]—M[i1]—.| | | |LP[i0]–W[i0]—W[i1]—RP[i1]| | | |.——-N[i0]*–N[i1]*–.The optimal solution is given by:
| .-------M[i0]---M[i1]---. | ---N[i0]---N[i1]--- | | | | | | | = SVD of LP[i0]--W[i0]---W[i1]---RP[i1] | | | | | | .----- --.
- Parameters
psi (
MPS
) – The state to whichoptions (dict) – See
VariationalCompression
.
Options
-
config
VariationalApplyMPO
¶ option summary Whether to combine legs into pipes. This combines the virtual and [...]
init_env_data (from Sweep) in DMRGEngine.init_env
Dictionary as returned by ``self.env.get_initialization_data()`` from [...]
lanczos_params (from Sweep) in Sweep
Lanczos parameters as described in [...]
N_sweeps (from VariationalCompression) in VariationalCompression
Number of sweeps to perform.
orthogonal_to (from Sweep) in DMRGEngine.init_env
List of other matrix product states to orthogonalize against. [...]
Number of sweeps to be performed without optimization to update [...]
Number of sweeps that have already been performed.
trunc_params (from VariationalCompression) in VariationalCompression
Truncation parameters as described in :cfg:config:`truncation`.
Level of verbosity (i.e. how much status information to print); higher=more [...]
-
init_env
(U_MPO)[source]¶ Initialize the environment.
- Parameters
U_MPO (
MPO
) – The MPO to be applied to the sate.
-
update_local
(_, optimize=True)[source]¶ Perform local update.
This simply contracts the environments and theta from the ket to get an updated theta for the bra self.psi (to be changed in place).
-
environment_sweeps
(N_sweeps)[source]¶ Perform N_sweeps sweeps without optimization to update the environment.
- Parameters
N_sweeps (int) – Number of sweeps to run without optimization
-
get_sweep_schedule
()[source]¶ Define the schedule of the sweep.
One ‘sweep’ is a full sequence from the leftmost site to the right and back. Only those LP and RP that can be used later should be updated.
- Returns
schedule – Schedule for the sweep. Each entry is
(i0, move_right, (update_LP, update_RP))
, where i0 is the leftmost of theself.EffectiveH.length
sites to be updated inupdate_local()
, move_right indicates whether the next i0 in the schedule is rigth (True) of the current one, and update_LP, update_RP indicate whether it is necessary to update the LP and RP. The latter are chosen such that the environment is growing for infinite systems, but we only keep the minimal number of environment tensors in memory.- Return type
-
post_update_local
(update_data)[source]¶ Algorithm-specific actions to be taken after local update.
An example would be to collect statistics.
-
reset_stats
()[source]¶ Reset the statistics. Useful if you want to start a new Sweep run.
This method is expected to be overwritten by subclass, and should then define self.update_stats and self.sweep_stats dicts consistent with the statistics generated by the algorithm particular to that subclass.
-
run
()[source]¶ Run the compression.
The state
psi
is compressed in place.- Returns
max_trunc_err – The maximal truncation error of a two-site wave function.
- Return type